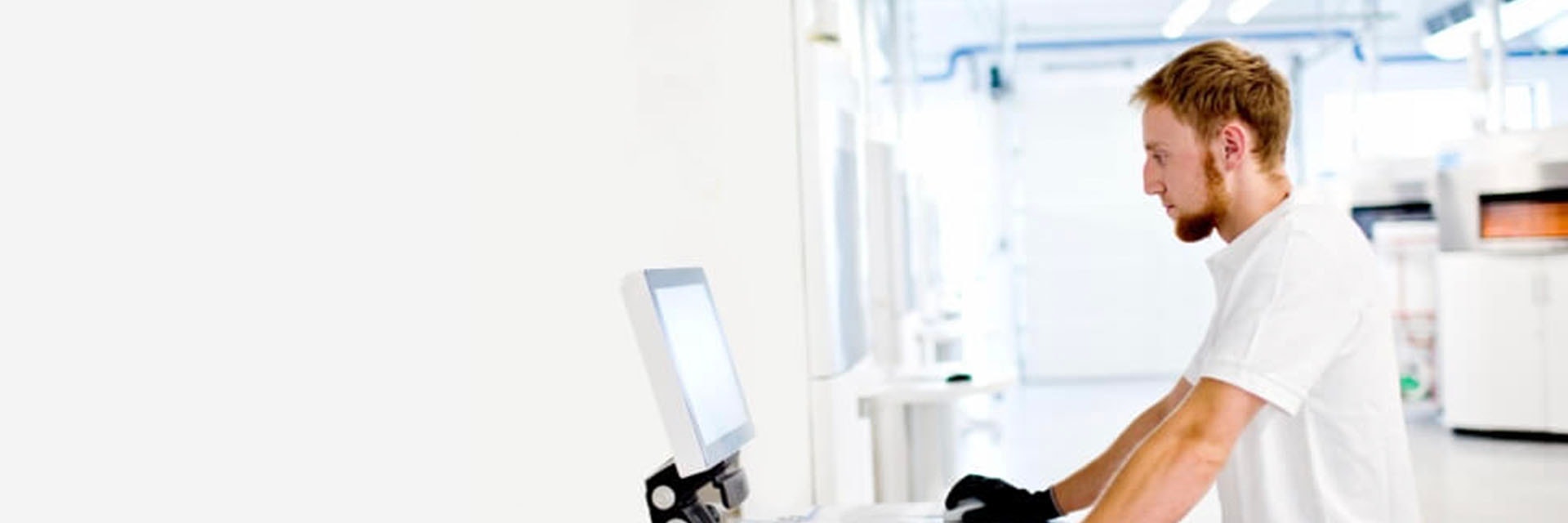
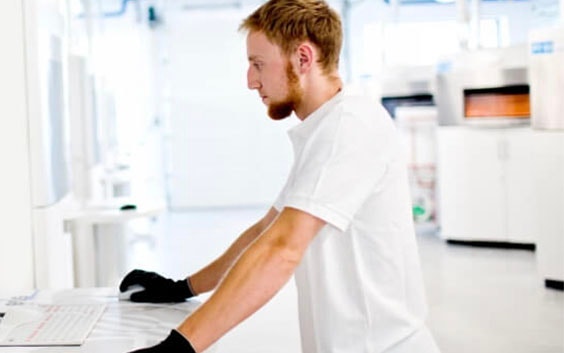
INTERVIEW
Applying Machine Learning to Layer Image Analysis to Reduce Costs and Increase Build Reliability
4 min read
At Materialise, our software solutions are often inspired by challenges the team faces within our own production facilities. A prime example of this is a software solution for layer image analysis. In this interview with Market Development Manager Gilles Claeys and Digital Intelligence Manager Jan Van Espen, we explore the difference this software will make in additive manufacturing (AM).
Hi, Gilles and Jan. Let's begin with the inspiration behind this solution. What was the challenge that sparked the idea for layer image analysis software?
Jan: In the period of 2015 – 2020, I was responsible for the team establishing our certified metal AM production at Materialise HQ. One of the things we learned during the validation process is that looking at AM layer images was crucial. Several engineers were sifting through thousands of layer images to search for errors. We found this work costly, frustrating, time-consuming, and repetitive.
Therefore, we almost immediately kicked off an initial automation project with the Materialise software team, which has — after more than 5 years — successfully lead to this Layer Image Analysis solution.
In my current role as Data Intelligence Manager, our team focuses on providing innovative solutions that answer customers' challenges in the field of AM quality control. All this is leveraged by combining our strong in-house AM process and software expertise in a single team.
Why is it necessary to conduct an analysis of layer images? And what is the process like when done manually?
Gilles: When Jan's team combed through the layer images for our in-house production, he noticed that the layers show many great insights into the quality of your build. Are there critical defects in the build? What is the root cause of these defects? Should we even spend time and money on post-processing the build? Layer images can provide an answer to these questions so AM users can fine-tune their processes to reduce waste, save costs, and ensure consistent and reliable results. So it's ideal for any production that wants to optimize its resources — and especially useful for industries like aerospace and healthcare where quality needs to be guaranteed.
But the current process is too slow, too time-consuming. Often there is no other choice but to review each individual layer within a build via images taken from metal 3D printers' cameras. Depending on how big your build is, it could take more than a couple of hours to review all of the images. And, of course, the process is prone to human errors. People interpret the data differently, or their eyes become tired, and they miss an error.
What is this new solution? And how does it solve these challenges?
Gilles: Layer Image Analysis is a module that uses artificial intelligence to examine layers snapshotted from the cameras on 3D printers. In a matter of seconds, the tool scans the layer, identifies errors, and visualizes them on each layer image and on the 3D model of the build. The user can then get a summary of defects in the build or review the errors in detail, color-coded by category, to conduct root cause analysis and pinpoint how they each affect the build. It also identifies errors that are not easily identifiable to the naked eye.
Jan: First of all, this clearly saves the user a ton of time. What could take hours for complex builds can now be done in the blink of an eye. It also gives extra users insights to make their production more reliable. Immediately after printing the part, using Layer Image Analysis helps you quickly determine whether the quality is high enough to move forward to post-processing.
There's also the possibility to look further into the details and metrics of each error — its size, for example. This is a great opportunity for those in highly regulated sectors to automatically eliminate any part with errors greater than a specified size, ensuring all parts meet certified standards.
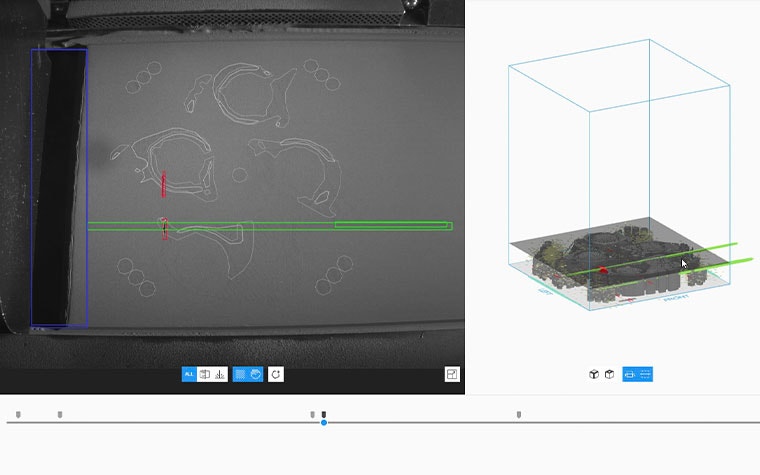
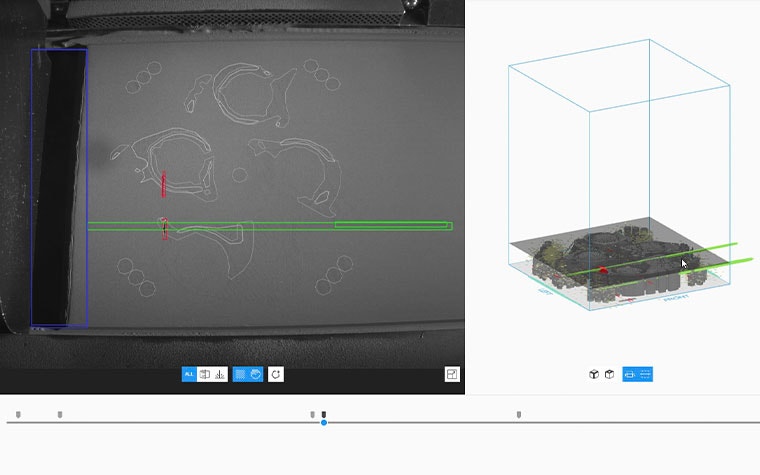
What are some other benefits that AM users can achieve with this process?
Gilles: Getting insights into your build quality is one thing, but another advantage we haven't touched on yet is the cost savings. Post-processing and nondestructive testing can account for 30 – 70% of the AM production costs in some industries. And when parts with errors nearly invisible to the eye slip through, a lot of money and time is spent finishing parts that will need to be thrown away anyway. With Layer Image Analysis, builds with defects that don’t meet production standards can be flagged automatically so engineers can review the part before passing it along to the next step — all in a matter of a few minutes.
Jan: There's a significant long-term advantage to using this process as well. Over time, users can collect these insights to improve machine performance. Say a machine repeatedly causes a mistake at the same layer during each build. Without automated analysis functions, it could take a long time to detect the repeat error and take the necessary steps to fix it. But by reviewing build analyses with Layer Image Analysis, users can sooner correct machine issues and ensure a more reliable flow.
What has been the response to this automated solution so far?
Gilles: We are collaborating closely with customers to develop this tool and are currently completing a testing phase. They've begun using Layer Image Analysis on their builds, and we've been working with them to review the layers and verify whether the automated results match the expectations. We're very close to completing this phase and having a product ready for launch.
Jan: One example is Airbus in the UK, with whom we've collaborated over the past few years, providing automated layer image analysis to help them further optimize their AM process and quickly compare the performances of their machines.
What can we expect next?
Jan: This is just the beginning. We'll be able to officially launch Layer Image Analysis soon, and we already have plans for future upgrades. One major step will be live process monitoring. This will flag errors as they form, allowing operators to pause the build or even make corrections, reducing waste from failed builds and improving machine productivity.
And layer images are only one way to get more insights into your build. There are still plenty of other data sources that can complement this to give an overall view of your build quality and make a significant difference in additive manufacturing.
Share on:
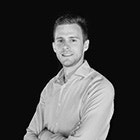
Biography
Gilles Claeys
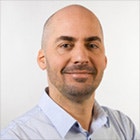
Biography
Jan Van Espen
You might also like
Never miss a story like this. Get curated content delivered straight to your inbox.