PODCAST
Medical 3D Players: How Can Computer Simulation Save Lives?
Discussing mass personalization in healthcare — because one size fits no one
- Subscribe:
- Apple Podcasts
- |
- YouTube
- |
- Spotify
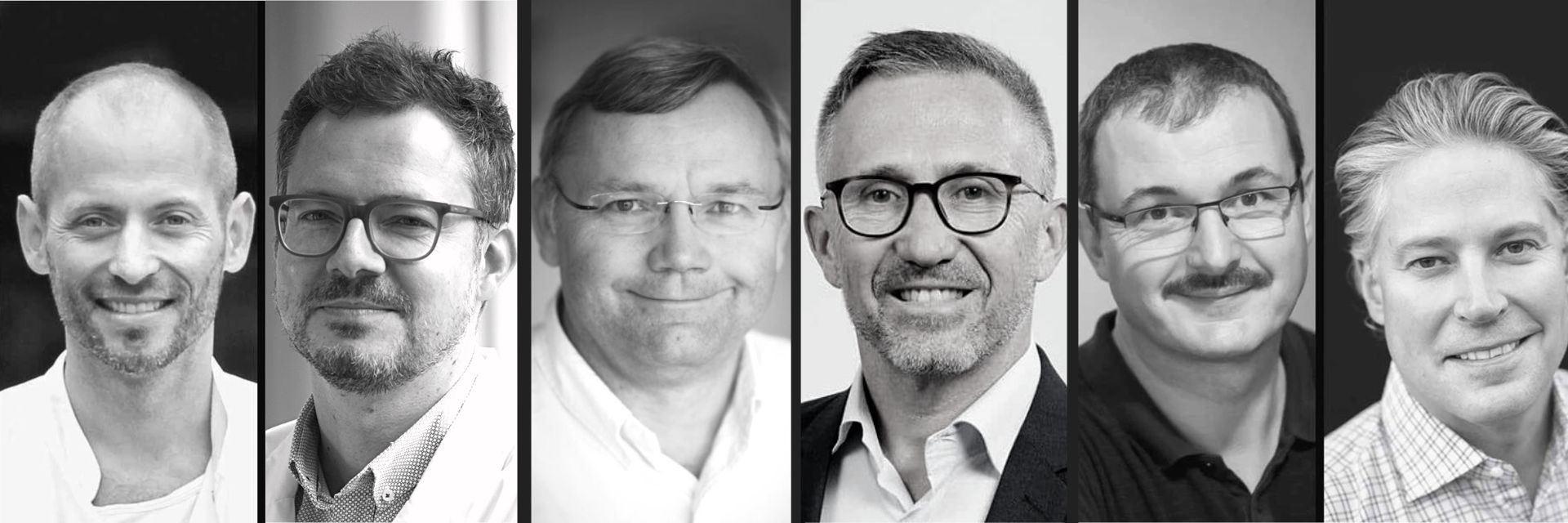
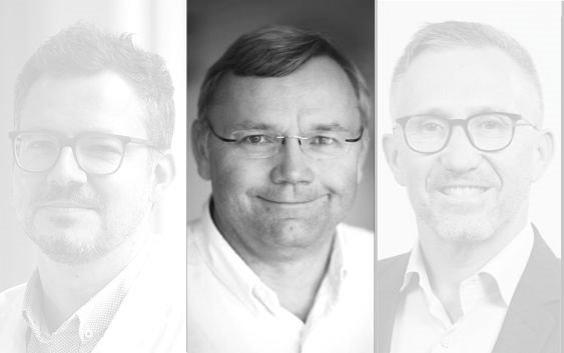
Uncover the latest medical advancements and challenges in 3D technology. Hosted by Pieter Slagmolen and Sebastian De Boodt from Materialise, this podcast examines key developments with experts in the healthcare industry.
In this episode, you'll be surprised to learn why computer simulations are easier to develop for a patient than an airplane. We also cover how digital twins, when combined with actual patient trials, can lead to quicker adoption of life-saving medical care. Hear Thierry Marchal, Industry Director for Healthcare Solutions at Ansys, explain why the medical industry, including 3D and personalized care, needs to collaborate to transform healthcare faster.
- Subscribe:
- Apple Podcasts
- |
- YouTube
- |
- Spotify
Read the full transcript
Sebastian De Boodt 00:00
Welcome to the 3D Players podcast, where we explore personalization in healthcare through advancements in 3D technology. We'll talk to leaders championing more predictable and sustainable patient care. I'm Sebastian De Boodt, and I'm joined by my co-host, Pieter Slagmolen.
How do you know which personalized approach will lead to optimal patient outcomes? Perhaps the key is in predictive planning through computational modeling and simulation. At least, that's what you'll find out today from our guest, Thierry Marchal. Thierry has been with Ansys for over 30 years and is now the Program Director of Healthcare Solutions. He's tirelessly evangelizing and working towards the adoption of in silico methods and the digitization of healthcare. And since 2016, he's also been influencing policymakers as the Secretary General of the Avicenna Alliance. In other words, if computational modeling is our crystal ball, then Thierry Marchal is one of the best fortune tellers out there. Welcome, Thierry. Maybe for anybody not familiar with computation, modeling, and simulation, can you explain what it is?
Thierry Marchal 01:12
Well, definitely, that's a technology for the future. But also, from the recent past, we are creating a computer model. A computer model is just a numerical model of what's happening in reality. And this has been used across the industry for over 50 years. Why do we do that? When you're doing the experiment, we have the real person or the real airplane flying, but you don't have all the information over there. When you're creating a computer model, you've got all the information, the stress, the velocity of the fluid, and so on, everywhere in the computer model, that you can derive from the experiment, but you can collect a lot of more information on top of that, that means that you can bring in a lot of variation. What if, in fact, the patient is not like you, Sebastian, a man, but a woman? It could bring in some difference. All that gives us more insight about what could be done...
Pieter Slagmolen 02:08
Thierry, one of the things already mentioned — airplanes — is that technology already applied as opposed to healthcare. Can you give some examples maybe on how computational modeling today is already impacting our lives in other areas than healthcare?
Thierry Marchal 02:19
Well, it is impacting life everywhere. For example, the fact that we've got a good temperature here, the airflow for air conditioning, has been used by simulation. Today, the airplane has been designed using a computer model, from Ansys and from all the tools, for the last 50 years. So that means that every single plane still flying today will have been designed with simulation. Or take the car. The fact that in order to make sure that whenever you crash, like with the ice on the road yesterday, all the inhabitants of the car will be in a safe condition means that we need to do a lot of analysis. Rather than crashing this nice car, which is very expensive, people are using computer models in order to see what's going on. That doesn't mean that we will get completely rid of this kind of testing. That means that we can do a lot of tests on the computer. But thinking about the computer, your cell phone, all that’s needed to be a model extensively with simulation, whether we are sending the right energy — like whether they will be not sending energy in your head when you are you're giving a call — all these kinds of things could be a model.
Also, we're talking a lot about energy and sustainability right now. Climate conditions, or can we move from fossil fuel to this kind of a renewable energy? That's a major challenge! Or can we change from a combustion car to an electric car? Well, we need one century to update the combustion car to the level that we've got today; we've got only ten years to make sure that the electric car will be essential. That means that we need to do all these kinds of simulations to accelerate the process. At the same time, reduce the cost to go to market. So, that means, this technology that we are discussing today has been around for over 50 years.
In fact, Ansys has been created 52 years ago. It’s nothing new. And people are sometimes a little bit hesitant to say, "Okay: simulation for healthcare. It’s a nice colorful picture, but can I really trust it?" I used to say, "Okay, if you don't trust simulation, don't fly, don't take a flight anymore. Stay away from your car, stay away from your cell phone, because all of that has been using simulation for a long time." That doesn't mean that we can completely trust simulation. That means that we can try the technology. But, we need to continue to challenge the model.
Pieter Slagmolen 04:33
The things that you say are interesting in that you said, "Okay, we want to avoid having to crash all cars." To predict what's going to be the best design of a car, for example, you can put this in a computer and then model that. I guess that there's a parallel in healthcare. Right? So So, if you draw that parallel, why why is this kind of technology relevant in healthcare, and then maybe more specifically towards personalized care in scalable personalized care?
Thierry Marchal 04:55
Well, as soon as you ever create a computer model, let's take a viewer, Pieter. Well, you create a computer model of yourself, suddenly, you're not feeling well, that's a way to say "Okay, what's going on with Pieter?" He was having too much wine yesterday evening. We can ask this kind of personalized healthcare, what do you need? Take a more serious example, if you are diabetic, and therefore you need some kind of insulin, what is a dose of insulin that we need to inject?
You might be forgetting that we can model your body in such a way that together with a sensor, we can [create] a complement, a kind of digital twin; the digital twin will be in parallel with the physical twin, and the digital twin is continuously connected over there, so the physical twin will take some measurements that are fed into the digital twin, and the digital twin will be able to predict. That’s the goal of the simulation and the computer modeling. Simulation here is being able to predict what will happen in a certain condition depending upon some event. And we see that, for example, you are eating a good lunch or a good dinner, we can predict that indeed, you will need to get more insulin, “Let's measure glucose in your blood.”
Considering that we know whether we can introduce some more insulin into your body so that you will feel comfortable. People are even thinking, "I've heard a few years ago that maybe we can connect this kind of wherever insulin pump together with Google." Google will detect that you are arriving in a famous restaurant that you like very much. And so Google and this wearable insulin pump meter, if Pieter wants to go to a very good restaurant, let's then pre-inject some insulin so that he will feel completely comfortable. That's the kind of future that we can aim for which is becoming a reality. Of course, there are a lot of things to consider, because privacy of the people is also important. We always need to coordinate with the regulatory environment. But the technology needs to be ready, and it is getting ready.
Sebastian De Boodt 07:03
That's very interesting to see. And not sure if I'm ready yet to trust a system to inject a medicine as I'm going into the restaurant, but I think we'll be getting there. And you know, I think it's probably a matter of time just to get used to the idea.
Pieter Slagmolen 07:15
Some self-control is still something I would like...
Sebastian De Boodt 07:22
Now, I could indeed see, if I'm understanding correctly, computational modeling and simulation, basically, you know, allows doctors to try out, you know, different scenarios in how they could treat a patient before really treating them which sounds very, very powerful if we could do that. Where are we now when it comes to applying the technology in day-to-day practice? Do you have any examples of how this has been impacting personalized care?
Thierry Marchal 07:46
I would put two things separate. Indeed, there are doctors that can take advantage of the simulation, but there is a lot of discussion. We'll come back to that later. On the question of liabilities, if the doctor is trusting simulation results in order to make the decision. And by the way, the doctor is always the one making the final decision; it’s never the result of a simulation. But where is the liability? Is a simulation result influencing the doctor? That's a big open question right now that we are still discussing with authorities, but we need to make sure that the results are validated enough that we can answer that. But there is another element where simulation is used extremely regularly: in the design of this kind of device, whether it is a sensor to make a diagnosis, or whether to make some kind of treatment.
Let me take an orthopaedic example. If someone has been impacted badly, unfortunately, maybe in Ukraine, right? Also, in any kind of car accident as well. It means that we need to replace part of the skeleton with some kind of part, which can be 3D manufactured. That could be a very good idea here to say "Okay, well, I want to really personalize the implant to the people in front of us." We can take medical imaging of the patient in order to find out what should be the shape that we want to, to introduce and 3D print the shape. We can model the kind of 3D shape in order to make sure that this will be good. But that means that we will be implanting a new device in the patient without any testing. That's not very much in line with the FDA, the Food and Drug Administration, or the European Authority, and so on.
Now, let's think a little bit differently. Let's imagine that you've got a bad accident. We scan you very quickly, we design the shape of the implant that we want to do. And then we are performing a few tests in order to make sure that this kind of implant will react correctly with the body, there will be no problem with any kind of impact, the structure, the fatigue will be good enough. So that means that before making any kind of implant, we are collecting enough testing, enough information to have the same certainty that some things are targeting going through a clinical trial. But that is enough to move into this area.
Those are some examples of today's application of simulation; we can always predict what's going on for a given patient. And we can fully personalize this kind of approach. Later on, the clinician comes, and the clinician is willing to do that. If I may, let me take an example from Dr. David Hoganson from Boston Children's Hospital. He was getting a little girl, her name was Ali, who was presenting in one of our conferences. Ali has got a major heart malfunction, the blood was not going to the lungs anymore. She's got about one year to live, very sad, she was 11 years old. So, he was counting the thoracic cavity of the patient to analyze the blood flow and created a computer model. To some extent, it was relatively simple. "What's going on? If I'm doing a bypass, where will the flow go?" First, it confirmed what he was learning from the surgical training, the flow will go mainly to the left lung and not to the other one. So he was saying, "Okay, let me play a little bit with this geometry, this virtual geometry. Let’s see the digital twin of little Ali and see what's going on. You find a way in which 54% of the blood was going to one lung, 46% to the other lung. Okay, that's good, let me follow this kind of surgery." And he was very surprised because not only the surgery was working well, but on top of that, this little Ali, who should have stayed for one month in the hospital, after one week, she was running everywhere. They were telling her to go back home — saving a lot of money for the insurance company...sorry to talk about money, but this is also part of it. And a few years later, little Ali kept growing and playing with everybody. That’s the kind of real impact. But again, we're to make sure that the surgeon fully understands what is the role and limitation of the simulation so that they can reapply it.
Sebastian De Boodt 11:55
And I think it's a very strong example of the value that it can bring. And you you clearly point out that the surgeon needs to understand the possibilities but also the limitations. How do you explain that? Because, I mean, it's very advanced, what you're doing, and it's typically not what any physician has been trained for. So how do you help him understand the limitations of the technology?
Thierry Marchal 12:16
Well, I've gotten the chance to speak several times with a clinician in different hospitals, including here in Belgium. These people are very smart but don't have the time to learn or to use this kind of solver because they're fully dedicated to saving lives, and they just don't have time to run the solver. Well, these people are ready to trust. If you show them that indeed, this is not just a colorful picture, but you can read through these kinds of things. And I was showing the Navier Stokes equations to the technician telling them, "okay, you don't need to understand that, you need to understand what we are solving — mass conservation, energy conservation, basic laws of Newton." I was also showing, and I think the same message, in front of the European Parliament. They understand this kind of thing, they understand the basics, that we can help them. And after that, to some extent with simulation, you can see what they cannot see.
We used to say that simulation gives you superpowers, you can make the invisible visible. When, coming back to Dr. Hoganson, you can see where the blood flow is going on. That's quite easy. And provided that you can trust this kind of result of a simulation that we'll look at that just like is a way of manipulating different kinds of elements and use their common sense of "Okay, where can I go? What do I do? What do I need to do?" We don't need to teach the clinician to some extent; we need to work with them in such a way that we are developing some application that’s very easy for them to use very quickly. And after that, they can move on.
Pieter Slagmolen 13:42
That's interesting because, in a way, that is maybe the role of industry in making this technology more accessible and more scalable, as in finding ways in which we can translate what is intrinsically complicated. Translate that into solutions that are easy enough to understand. For a physician maybe to have more visual aspects to it that translate more closely to their patient and things that they do understand.
Thierry Marchal 14:05
Well, we tried that in the past; it was a complete failure. Because we show a simulation, we're very proud to show them, here's what you have to do, we're very excited... and then never use it! Because we were not answering their question. What we found out what is working very well is that we are at the service of all these clinicians. We are showing them the power of simulation, and then we ask them, "Okay, what do you need? What are the problems that you're facing? What are your challenges?" The only way to design a clean-cut application that will be valuable for the clinician is to do it with them. And by the way, not to tell them, "Okay, come to our office and we’ll discuss that." I tried that as well. And most of the time when you've got a meeting they need to come to, they've “got an emergency surgery” and all those kind of things.
What we found is that by giving them pieces directly in the hospital, that’s where it’s going on. Whenever the clinician has a problem and they are both in the room where they hear the engineer say, "Okay, here's my plan, how can we solve that?" And we keep discussing with them, the clinician defines and designs what they need, what will be interesting. And by the way, sometimes this is something very basic. We used to solve some things that were very advanced, we're very proud of that. Very nice, but they don't care about that.
Another example: we were working with ophthalmologists. These ophthalmologists, in order to treat cataracts, they need to make some small cuts in the eyes. The problem is the length and the depth of the cut. If you're doing that with simulation, it’s based on average eyes. If you are average, perfect, you have perfect vision after that, after the one surgery. If your eyes are not average, that means that you need two or three surgeries, or you still need glasses. Some people in Switzerland design software or clinical applications in order to scan the eyes. And based on the scan and the mechanical deformation of the eyes, during the laser treatment they define exactly what will be the vision after some specific surgery. You can start to optimize the start of the surgery. Based on that, you can give some guidance and good insight to the surgeon — all that has been done with the ophthalmologists. When you look at the interface of this tool, you also say that doesn't look very good. It looks very complex. No, it's exactly along the line of what ophthalmologists are used to doing.
Along this line, we recently got the interview of a 76-year-old guy used to driving his car, watching TV, reading a new series, or doing a tiny bit of work. He was not able to do that unless he had five different pairs of glasses. We did the surgery, and he can do all the things like what he was doing in the past. If we are not working end-to-end with true dedication, you're wasting your time, and more importantly, you're wasting their time.
Sebastian De Boodt 16:48
That collaboration sounds very similar to what drove the adoption of 3D printing in hospitals, and where it's also been... because hospitals taking this inside the hospital means that you have this good collaboration between engineers and clinicians... all sorts of meaningful applications are suddenly arising, which is great development. Now, it doesn't always scale that well. And, if you you need to always have you know, somebody very technical and probably highly educated alongside a physician, what does it take to then scale computational modeling? And do you perhaps have any success stories to share of applications that have really managed to scale up and reach really more patients?
Thierry Marchal 17:30
There are some of them. Indeed, one of the clients when I was in Utah, we had our own engineer in the hospital at the beginning to design these elements and to find out what is a reasonable computational time (and we are talking about minutes, sometimes seconds). Why? Maybe the surgeon will do the surgery after a few days, but the focus of the surgeon is only for a few minutes on a given patient. So, we need to get all the results at that time. After that, if we want to deploy that, certainly we need to have this kind of user interface, which is familiar to the clinicians, ophthalmologists, cardiovascular surgeons, and the right time and access to the right computational power, like the cloud (provided that we can do that outside of the hospital).
But I would say that the biggest problem right now is the adoption. Clinicians are always a little bit hesitant. They don't know what it is. So even if we have a very good solution, that means that they don't know — they don't have time to do the communication, telling them that we've got excellent success stories, bringing them to try to do that. Even the young surgeons, the younger doctors, might be open; others might see that and think, "oh, this was his will replace me, so I don't want one." They’re fearing this kind of thing. So we need to make sure that everybody is feeling comfortable, that we are bringing them additional insights so that the patient, with less risk of patient complaints... the patient coming back to him... and possibly to treat more patients at the same time. So right now, this is one of the key elements.
The other thing, as I was mentioning, is the liability. If the clinical application is giving you some result, of course, this is the clinician’s decision, but let's imagine that a patient is not getting some acceptable result. The patient might be complaining. And the clinician might be saying, "okay, but I was driven by the technical application." What is the liability? That's exactly the same for the autonomous car right now. If there is an accident, whose responsibility is it? The car manufacturer? The driver? The software developer? That’s not clear right now. I was discussing with the European Commission a couple of weeks ago. Indeed, we need to make some progress. That doesn't mean that the software developer has no responsibility. We just want to know, what do we need to demonstrate to show that we've done all our own work? Otherwise, it will be very difficult for groups like counties to say, "Okay, I will be investing a lot more money in healthcare because there is a big market opportunity" if the research has a big risk. So, we need to clarify the dilemma. We need to realize that this is a software company; we are facing competition between different industries. Should we invest more in automotive, in aeronautics, in healthcare, and so on? We know that there is a big market for healthcare; we know that we can really impact people, but we have to make sure that everybody will accept that, clinicians, but also the patient themselves. And later on, that there is no major risk if we are going there.
Pieter Slagmolen 20:29
So the elements that you bring forward to scale are, obviously communication and making sure that everybody understands what you're doing. And then evidence speaks for itself, I think, and obviously proving safety of the approaches. So how does that then impact your company's strategy? If you think about Ansys as a company, how does it impact your strategy in trying to make this adoption and broader adoption a reality?
Thierry Marchal 20:50
One of the key elements is to work as part of an ecosystem. If we are working alone, there is no chance that we can succeed. So, we've got a larger ecosystem with many academic hospital regulators. We are speaking regularly with EMR, the European Medicines Agency, with the FDA, and all these elements. We are collaborating with them, but also with the other software companies like Materialise, with other hardware companies. For example, in the future, you all will require a lot of simulation. Where can we find simulation in the cloud? We are working closely with Microsoft, with Google, with Amazon, in order to find the right way so that we can transfer the data, personal data, so we need to make sure that everything is fine. And all this data privacy will be properly protected, we need to take advantage of the European Health Data Space that has been recently created. So those are the elements that we are going to work with as part of an ecosystem; working with the key stakeholders, medical device companies, pharma, because pharma is taking advantage of that and it's not going quite well with these kind of clinicians, the academic people,
Pieter Slagmolen 21:58
One of the aspects that you didn't touch on is the, I would say, validation of some of the technology. Because indeed, you're working in an ecosystem, making sure that you provide safe cloud access, and so on. I think those are very crucial aspects in driving further adoption. But, in essence, the core validation of the technology in itself is going to be a big aspect of that as well. You have strategies that you can follow to make sure that simulation and modeling align with reality. Do you have a success story to share where you can demonstrate that it's well-aligned with reality?
Thierry Marchal 22:28
While validation is one of the most fundamental elements of healthcare (and simulation for healthcare), we are talking about two kinds of validation. First, we need to validate that compared to experimental testing, you're getting the right result. But what is even more important is clinical validation. Will the outcome, that we will see on a patient in the hospital, be the right one? That's why I'm talking about this ecosystem, the only way to have this kind of clinical validation is to work directly with the clinician, up to the point that we need to regulate all that. Validation is one of the key elements. And we were working with ASME, the American Society of Mechanical Engineers to define, if we are willing to use a computer model, we say “digital evidence,” the result of a simulation for regulatory approval. Meaning that rather than doing a lot of clinical trials, we can create some kind of digital evidence result of simulations before the clinical trial — very important. That means that we are communicating a lot of evidence before implanting some kind of device into real patient. This is much more ethical.
But the key question is how do we do the verification and validation? Verification means how do you solve the equation properly? Validation is how do you make sure that what you model represents reality? So with the ASME, we've been working for the last ten years in order to create the ASME V&V 40 (which is verification and validation for medical devices). This element will define in every single step, how can we make sure that we are verifying the solver that we are creating and how we validate the model, what will be the risk associated by this model. I can tell you that validation is maybe taking ten times more than the development of a new model because you can validate it in some specific condition, everything is working well. The power of simulation is that you can do that for a very large number of cases. And along this line to show that this is widely accepted.
I remember that in 2018, Medtronic was presenting in front of the European Parliament, an example where they create a computer model of the heart using a designer and a computer model of the pacemaker. They implanted the pacemaker into the heart into the computer model. In parallel of that, they were also doing some clinical trials, an extensive clinical trial. And by the way, the computer model was giving a result very similar to the clinical trial. They were presenting something older to the FDA, it was in 2017, the computer model and the result of the clinical trial. The FDA was very pleased with the situation and accepted the result, the digital evidence, the result of the model together with the clinical trial. Of course, the FDA had an additional question, what's going on in this specific condition if the patient is like that? Medtronic was asking, "okay, considering that you have accepted the computer model that we've used and validated with a clinical application, can we answer your question using the computer model?" And the FDA said yes. The result of that is that rather than doing a new clinical trial, an additional clinical trial, to answer only the question for 256 patients — the total cost could have been 10 million euros plus the time — would have taken two years to do that. The FDA was saying, "yes, indeed, you can use your computer model." After two weeks, only two weeks, they were getting all the results coming from the computer model to present that to the FDA. The FDA said, "okay, we agree on that." Well, you can say: "okay, that's good." For Medtronic, they were saving $10 million. The real story was the product was released two years in advance. That means that during these two years, we were treating 10,000 patients. 10,000 patients get this new implant with the new pacemaker. That means that 10,000 patients were benefiting from our new more advanced technology that’s better for the patient, rather than just using the old element.
So that's where this kind of simulation, using tools that we validate quite extensively before releasing the tool, but it's also validated by the clinician and by the regulatory authority, with the goal to accelerate medical innovation. That's one of the key elements that you were talking about in personalized healthcare earlier today. The reason to do personalized healthcare is that, for me getting a little bit old, I've got some excellent healthcare for my children and grandchildren. Later on, there is no chance that they will have the same quality of care that I enjoy if we are not changing things. Look at that the cost of healthcare, today it’s $9 trillion every year. It increases exponentially. If we don't do some things to personalize care, the quality of healthcare will go down. So that's a major responsibility that we've got here as part of this global ecosystem: we change the situation in such a way that our children and grandchildren will have better care than we've got so far.
Sebastian De Boodt 27:27
And is that you know, why you're also part of the Avicenna Alliance? Like is that one of the goals to really push this on the agenda of the policymakers so that it accelerates? Can you explain to us a little bit, what the Avicenna alliance is all about?
Thierry Marchal 27:39
Yeah, and that's a very good question. As I say, we need to be part of an ecosystem, we need to join forces in order to make sure that we can really change the situation; join forces between medical device companies, between pharmaceutical companies, between software companies and all that is done in a pre-competitive environment. The Avicenna Alliance is a Belgian organization, a not-for-profit organization, with the only goal to advocate and regulate the adoption of in silico method. That means that there are several competitors in terms of software developers but also medical devices. Groups like Boston Scientific, Medtronic, Stryker in the orthopaedic, Johnson & Johnson, and so on. We are all there to find out. Okay, what are the different challenges? What is AI for in silico, for example? How can we engage with the FDA and meet with them every month? How can we speak in front of the European Parliament? How can we engage with the US senators? And by the way, when we met with the US senators, when we were showing them the value of simulation, they quickly understand the value that it could have. They wrote to the FDA to say, "Okay, you need to develop some kind of digital twin, you need to adopt this kind of in silico." And I can tell you that the FDA was very pleased because they were receiving more revenue in order to push that. So as part of the Avicenna Alliance, one of the key elements is to join forces so that we are bigger and can influence different people. One of the key elements is that 50% of the Avicenna Alliance are from different academic groups, like KU Leuven, like University of Liege in Belgium, but like the most important universities around the world we need to combine this kind of research and business activity, with the goal to accelerate the development and innovation in healthcare by using these kinds of in silico methods.
Sebastian De Boodt 29:29
We are recording these episodes in December 2022. And the world just got overthrown by these Open AI chatbots. Now, both modeling and AI have the objective to predict the future and one does that through deep understanding of reality. That's what you're trying to achieve while the other is really just about, you know, huge volumes of real-life data without really caring at all or understanding the underlying dynamics. Now, we can't deny that the impact of AI has been exploding over the past years, do you actually consider modeling and AI as competing technologies?
Thierry Marchal 30:02
Well, they're not at all competitive, they are complementing technologies. AI is part of in silico. In silico is anything using computers by definition, and AI is part of in silico. On the other end, AI is using any kind of data in order to be able to predict what could be there, what could be done, and to give some kind of guidance. So, it could be real-world evidence, some things that the observation of what we are doing, but why not use simulation together with this kind of digital evidence, especially if no observation has been made so far? I'm thinking about this kind of orphan disease, and therefore the fact that we didn't have enough patients to do that. AI is taking advantage of simulation and results in kind of digital evidence to complement all this real-world evidence that they've got with one of the big advantages that the digital evidence has to go through a lot of validation. That's one of the elements that we were discussing earlier. But that's sometimes much better than any kind of observation which have been made, and for when we don't have enough confirmation that this is good quality.
On the opposite side, simulation is also taking a lot of advantage from AI. Well, for example, when you want to combine a model of the human body, but you don't have any physical or mechanistic model to show the evolution of a pathology, well, there is no way to move forward. Unless, if you have some kind of real real-world evidence, and AI extracting all this real-world evidence, allowing you to create this kind of model for the evolution of the pathology, suddenly, you can combine all of these elements. The advantage of simulation is that you've got a mechanistic model and therefore you can understand, why is it happening like that? On the other hand, AI is taking advantage of all of these elements not just related to the digital evidence. So, I think that there is a very nice complement for that. And I would recommend this whitepaper that we've recently published as part of the Avicenna Alliance, about AI and in silico technology.
Pieter Slagmolen 31:58
One thing that that triggers me in this discussion, as well is, how do you look at the role of “explainability” in between what AI is doing, and what computational modeling is doing (which the second is intrinsically more explainable than AI), is what is the impact on really bringing solutions to the market then?
Thierry Marchal 32:17
Well, that we are complementary, we cannot explain everything, but we can take advantage of all the elements that we are seeing. Along this line, let me take the example of European project Premade, which is in collaboration with different elements and this is part of it: the goal is to being able to predict the evolution of a tumor, a cancerous tumor in the abdomen of a teenager. Anything related to children is always quite important. Well, we’ve got very little information about what will be the growth of the tumor. Based on some observation, they were able to give a model about not only the growth of the tumor, but the evolution of the impact of the chemotherapy agent that you were introducing. Using that, they published a model where they're able to predict, with relatively good accuracy, the model in terms of volume — something like 92 to 94% accuracy of the model — the way to not only predict the growth of the tumor, but also the decrease of the tumor based on the chemotherapy agent.
So right now, there is no competition. The reason why AI was so popular compared to a simulation: AI is a buzzword, we are talking about artificial intelligence, so always fun. What is helping quite a lot with simulation right now is a digital twin, because that's also a buzzword. At the end of the day, the only thing that matters is a patient because it could be any of us here. What can we do for the patient? And one of my key concerns, when we need to develop this kind of digital twin or virtual twin is that sometimes we could be thinking too far in 20 years, 30 years, and so on. That's good, to have a big vision like that. But one of my questions is what do we do for the patient today? How can we save lives with this kind of cancer treatment? We've just created, as part of the Avicenna Alliance, a task force about cancer and in silico oncology. What can we do, for example, for breast cancer and prostate cancer, which are the most popular ones, costing a lot of money, but we can face all that as well. In silico can bring a lot of solutions in order to activate this kind of numerical innovation. So, I think that these two elements are complementary. If we are thinking about the patient, first, we'll take whatever in existing technology will help us to achieve this goal.
Sebastian De Boodt 34:37
You're clearly not tired, evangelizing the technology to the world. I mean, it's great to see how much passion you still you still bring. If there is one thing that you still can realize in the remainder of your career, what would you like it to be?
Thierry Marchal 34:50
I think that the goal is always to make sure that we can save more lives. And that means that we can make sure that the next generation will benefit from what we were doing. One is to make sure that the quality of the healthcare will be better because we are bringing these kinds of in silico. And if I may, there is another thing which is important to me, and which is becoming quite important for the healthcare industry, both medical devices and pharma. We need not just to save lives, but also to save the planet. Sustainability is becoming something extremely important in healthcare, we realize that while we need to make sure to improve the care, sometimes we are putting too much carbon in the atmosphere, we are putting too much waste in the world, which in the end brings more patients to us.
And right now, we realize, all the medical device [companies] and some in the industry, realize that by using simulation, we can combine the fact that we can bring more safety that’s affordable to the patient. We can reduce the cost, we can go faster, and we can be more environmentally friendly. So that's why I'm still so passionate about simulation. This is not the uber solution here, but with this tool you can properly receive quite a lot of things and make sure that we can just enjoy life and enjoy all pleasure we've got while at the same time adding a good element. And quite often when my daughters come in to my office to say "okay, but why daddy are you working so late?" I keep telling them that together, with the entire ecosystem, all the people working with me, we can make sure that their life will be 10 to 20 years longer and healthier. Based on that, they tell me to keep working as hard as possible.
Sebastian De Boodt 36:20
It's a really nice answer, Thierry, and probably the right answer. I was hoping a little bit that you were going to say, I'm going to help Wout Van Aert win the Tour de France by you know, improving the simulation because that's also things that you guys are working on. Right? And I'm taking a very big side step now, but since we're here in Belgium, I thought, hey, you know, go for it?
Thierry Marchal 36:38
Indeed. Well, I've had a chance to work with this team and this guy is really fantastic. I was explaining to Wout Van Aert [renowned Belgian cyclist] that we've made a mathematical model, we can find out the best mathematical position on the bike. But at the same time, I was saying "Well, we have to make sure that you are comfortable on the bike because you are racing for fix, six, seven hours." He told me "Okay, don't worry about if that would be comfortable, give me the best possible position." Those are all the kinds of things that we were doing. Wout had some difficulty in some stages of the Tour de France; what he's doing is fantastic. Always very proud to receive some nice jerseys from him and to help him to do that.
Not just in terms of cycling, but for example, all the stadiums, where we’re creating this kind of computer model for all the stadiums, especially to model the propagation of COVID in the stadium. If you remember one of the first things in Bergamo was the spread of COVID in the old stadium that has killed quite a lot of people. Now we can model, and we use the Johan Cruijff stadium, a new stadium in Amsterdam which models the airflow and COVID propagation in the stadium, putting 30,000 people in a computer model, which is a kind of world record. We run a simulation using a CFD with six billion cells in the model in order to be able to predict this kind of propagation. So yes, sport is an excellent element in order to show the simulation.
Coming back to one of the elements that we were discussing, communication is essential. And thus the goal for sport is not huge business. But I was quite often on TV to explain how we can improve the performance of the athlete in a perfectly ethical way. By doing that suddenly people understand and the invisible is visible. If we show the airflow from Wout Van Aert to Jonas Vingegaarduniform and others, that means that they will understand how to put on their helmet. I'm talking very seriously, we are showing some dimension to these champions in order to explain to them how to use a different element, making the invisible visible. That means that people understand the value of simulation and we say, "Okay, yes, indeed, we need to do that." Whether this is for sport, for Wout to win the bigger road, but also for healthcare, and to make sure that all the clinicians, all the patients are aware, we will say "Okay, that's what I want to do."
Sebastian De Boodt 38:59
I didn't realize, indeed, that this is also really helping to bring an impact in what you're doing in healthcare space, but it makes all the sense. And it really ties back to one of the earliest things we talked about on the communication and making it really known.
Pieter Slagmolen 39:11
And I think for a lot of people it makes makes performance very tangible, right? If you draw the parallel with an athlete. Well actually what we are trying to do is maybe towards performance of a physician or a surgeon or maybe even a patient in revalidation, potentially, we're trying to increase performance there as well, right? so it's very similar in a way and that's interesting.
Thierry Marchal 39:30
It’s similar to what has been done in other industries for so long. That's why I'm always a bit annoyed when people say, "okay, but you know, the human body is much more complex than an airplane." That's not true when you see the complexity of the airplane. But I think that this company, this other industry, is not making an excuse that their device is so complex. And therefore it’s, “we cannot use simulation.” And we're coming with the point with, okay, let's take advantage of whatever is available. And that's what we should innovate along this line. That's exactly the same point before having a digital twin of yourself down to the DNA level might take another 30 - 40 years to go there. But using the technology that we've got today, we can make sure that we can treat some difficult situations. Possibly if you’re concerned about heart failure, and extending your life, that's what we want to do to make sure that more people can take advantage of that and keep living.
Sebastian De Boodt 40:25
Is there any clinical application in particular that you say, okay, that's going to be the first one where we really see it’s been adopted broadly, or the next one, what would it be?
Thierry Marchal 40:36
There is one which has been widely adopted from Heartflow. They are doing some fantastic elements. Well, the Heartflow story is very interesting. They invest quite a lot of money, a lot of time, in order to convince key people. And therefore, after some time, people realize that indeed this kind of computer model, very well validated, very well trusted, can make a big difference. I think that we always need to have this kind of example, to make sure that the other will say, "okay, why not after all? Why not going to do this example?" So far is still very difficult.
One of the key elements that I was discussing with KU Leuven then, like a kind of a flagship for all the universities, we have to make sure that all the students, especially all those in biomedical engineering, are trained in simulation. They need to understand the limitations of that. That's a little bit the goal to say, "Okay, everybody can have access to this solver. Let's work with them. Let's work with the teacher so that they've got the right curriculum." And the student when getting out of the university will know exactly what it is. We need to make sure to start with the young people in such a way that they will be bringing that. I'm also talking about medical students, making sure that the future surgeon, the future doctor will understand the value. Some people are opposed to the use of simulation; we cannot convince everybody. That would be a big mistake. Okay, let's try to make sure that everybody agrees on this: there will be some people not willing to do that, for whatever reason, no matter how much validation we are showing them. But if we have a good fraction of the doctors or the biomedical engineers who say "Okay, yeah, that's definitely something that we need to do," that will be enough to initiate the movement and to start saving a lot of lives. When we are doing that, I'm sure that everybody will just go along this line.
Similarly, when you say that simulation can be used before a clinical trial, in order to find out what will happen in the patient, before we are implanting this kind of device, people are saying, "okay, no way simulation can replace a clinical trial in the future because we need to do that with the patient." Okay, I don't mind that. Let's make sure that before doing any kind of clinical trial, we've got all the evidence that we can do on the computer so that we maximize the safety when we are doing the clinical trial with real patients. And guess what? Like everything that happened in the automotive, aeronautic industry, and so on, people will be doing more and more computer models. Until some time when people will ask, "okay, but why are you still doing some kind of physical testing which shows exactly what you get out of the computer model?" Let's stop the physical testing because they will be okay. Will that happen in healthcare? I don't know. But for sure, what I know is that before doing any kind of in vivo testing for animals, or for humans, why not take advantage of the computer, or digital twin on the computer? You can click as many times as you want: there is no problem for that. But real patients? We cannot even harm them. You have to make sure that everything is fine.
Pieter Slagmolen 43:42
And I think that's a wonderful note to end our episode today on, Thierry. Thanks a lot for joining us, we always end with a few key takeaways from the episode. And I'd like to do that here as well. And for me, I think the key takeaways are probably the fact that it's easier to create a digital twin of a patient than it is of an airplane. And that it's going to allow us to eliminate the need for car crashes to simulate what's happening to the patient between you and a computer, so that's interesting. The role of the end-to-end collaboration with clinicians that's really gonna make or break the adoption and scaling of any type of technical solution, which I think is a very good point that you make as well. And then one aspect that I found very interesting is the fact that using this kind of technology, modeling and simulation, can help bring innovations earlier to patients than with more traditional approaches. And that can only benefit these patients going forward, which I think is very exciting. So, thanks a lot, Thierry, for being here with us today.
So we appreciate your insights and sharing them with us on 3D Players. This is a podcast where we explore trends, insights, and innovations in personalized and sustainable healthcare. We're your hosts Pieter Slagmolen and Sebastian De Boodt. Thank you all for listening, and join us for the next edition.
Featuring
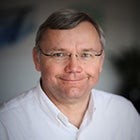
Thierry Marchal
Program Director of Healthcare Solutions, Ansys
About your hosts
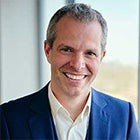
Sebastian De Boodt
Market Director, Materialise
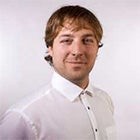
Pieter Slagmolen
Innovation Manager
Share on:
L-103195-01