EXPERT INSIGHT
How Will AI-Enabled Segmentation Boost Mass Personalization?
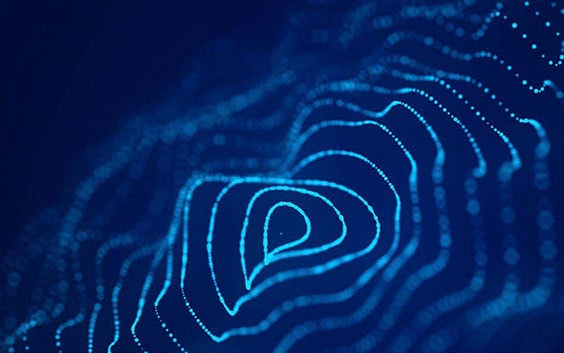
The segmentation of 2D images to create 3D reconstructions is an essential step to ensure accurate 3D analyses, planning, and printing. Automating this process for all anatomies could save time, increase efficiency and consistency, and ultimately help users scale patient-specific solutions by reducing the complexity and barriers for medical device companies and hospitals.
As the Mimics software turns 30, Materialise celebrates the launch of a powerful AI-based feature with the potential to disrupt bone segmentation procedures.
The challenge with segmentation
The process of creating virtual and printed models based on a patient’s unique anatomy was first achieved 30 years ago by Materialise CEO Fried Vancraen. This lightbulb moment led to the launch of the first version of Materialise Mimics two years after the company was founded.
Since then, this process has paved the way to uncharted possibilities in medicine, including creating 3D-printed anatomical models, surgical guides, and personalized implants — all contributing to more surgical predictability for clinicians and improved patient outcomes.
Over the last 30 years, Materialise has continuously invested in developing improved segmentation features within the Materialise Mimics Innovation Suite (MIS), which has become a reference in quality and reliability in the medical market.
Despite significant improvements over the years, manual and semi-automated segmentation are still a demanding task that requires time, skills, and expertise. That also means that the accuracy of your model is partially dependent on the engineer performing the task.
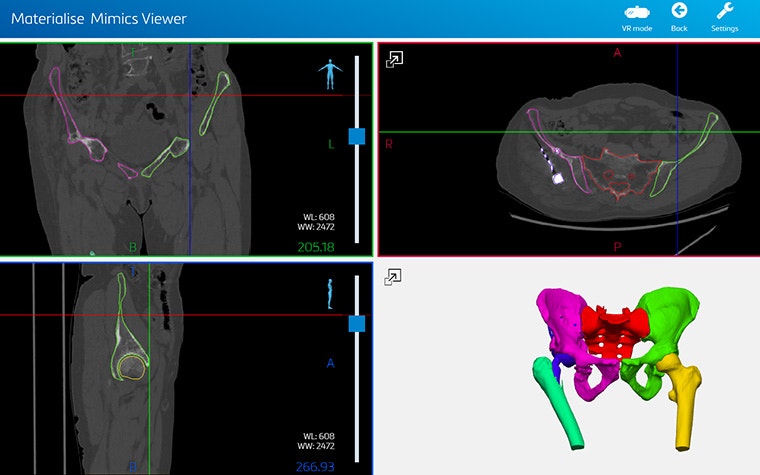
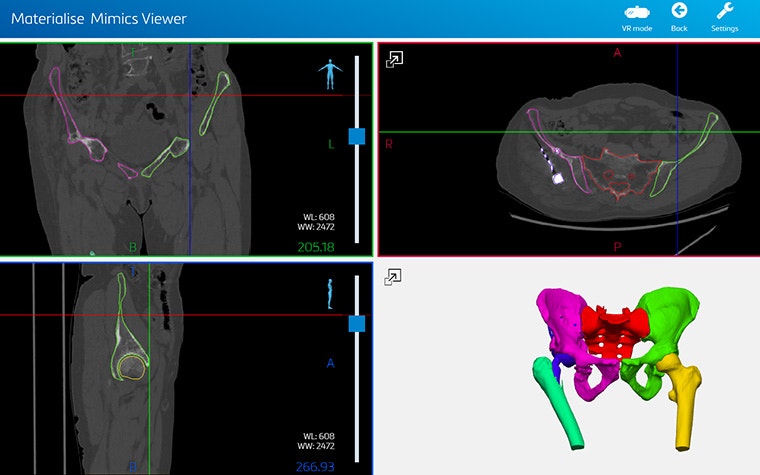
Fully automating this process could save time and increase efficiency, consistency, and accuracy.
With this in mind, our multidisciplinary research team of over 20 engineers worldwide, led by Research Engineer Sergiy Volkovych, has accepted the challenge to develop a fast and generic artificial intelligence (AI) solution for CT scan segmentation of bony structures. In other words, a solution that can correctly segment various anatomies and label different bones in just a few clicks and a couple of minutes.
What it takes to develop an AI algorithm
In machine learning, 80% of the time is typically spent on data preparation, so the machine learns how to do the work from examples instead of hard coding.
For the past two years, our research team has been collecting, analyzing, and preparing CT scan data containing healthy and defective bone structures to train and adjust a new segmentation model.
“Processing and translating all this data to train an AI algorithm demands extensive and intensive work involving deep learning, statistics, and classical image processing. Apart from this, we need to have this huge amount of data readily available, which is not always the case for many research teams trying to develop an AI algorithm,” says Sergiy.
The biggest challenge to overcome when creating this kind of automated solution is the variability in the anatomies posed by the human body. Not a single person is the same, and there is also variability caused by diseases, accidents, etc.
An efficient AI algorithm needs to be able to capture all of it and make the discernment between all anatomies independently of the bone situation. This discernment requires brain power, so Sergiy’s team put together the collected data in a U-net convolutional neural network that mimics the human brain.
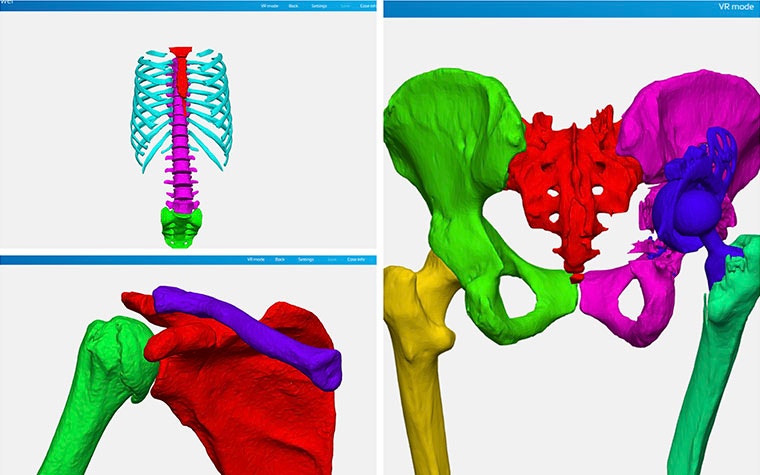
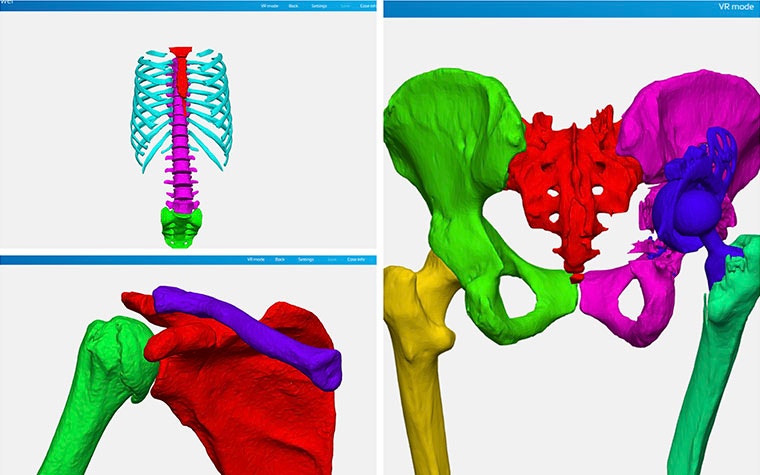
This challenging research resulted in a completely automated tool for accurate bone surface detection and segmentation launched with MIS 25. Impressively, it can segment and recognize 34 bony structures at once, labeling them according to their locations in the left or right part of the body.
How AI segmentation differentiates from current solutions
During an external validation of the MIS 25 AI tool, consistency in accuracy was praised as the main value driver. The second obvious benefit is the time gained and, therefore, the opportunity to focus on tasks other than segmentation.
Another important factor is the potential to save on training and equipment costs because the solution is available in a cloud system accessible from any ordinary desktop or laptop.
And last but not least, it is validated for clinical use and can immediately be integrated into existing clinical routines.
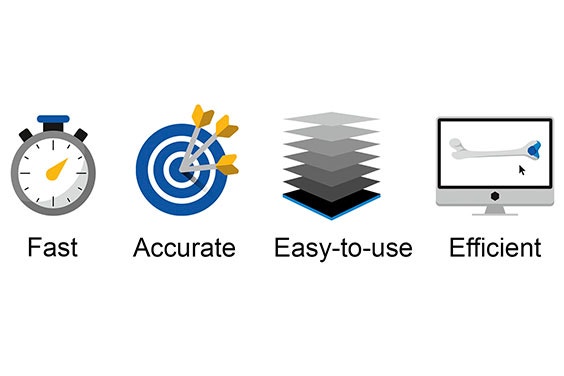
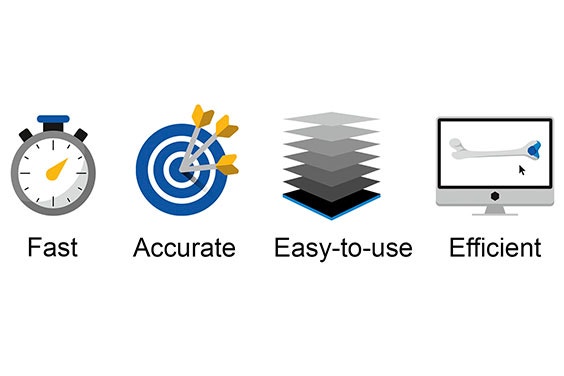
Breaking new ground in the medical field
As the market evolves, more and more customers need solutions that enable personalized treatments at scale.
The launch of the AI segmentation algorithm is a continuation of our mission of making personalized care more sustainable through process automation and better integration into the digital healthcare ecosystem.
The results achieved so far give us the confidence to say that AI is the way to go to scale mass personalization. And at Materialise, we will continue making the right investments to further progress in this field.
L-103061-01
Share on:
You might also like
Never miss a story like this. Get curated content delivered straight to your inbox.